Why Photovoltaic and Energy Storage Are Critical to Powering the AI-Driven Future (And How to Close the Gap)
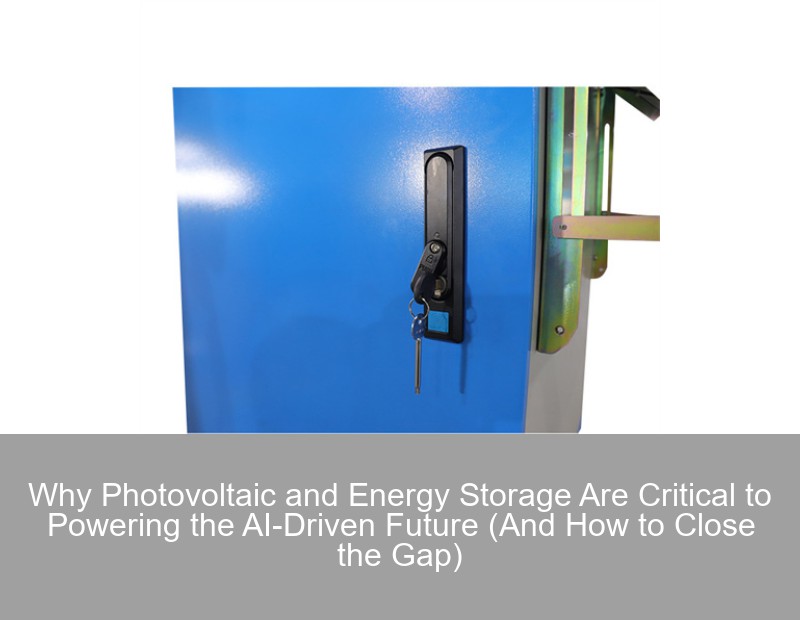
The $200 Billion Question: Can Renewable Sources Keep Up With AI's Insatiable Energy Appetite?
In March 2025, NVIDIA CEO Jensen Huang made a startling prediction at the World AI Conference: "Every 10% increase in global AI adoption requires a 15% boost in clean energy storage capacity." This isn't just tech executive hyperbole – Goldman Sachs estimates AI data centers alone will consume 8% of U.S. electricity by 2030, equivalent to powering 30 million homes .
Year | Global AI Energy Demand (TWh) | Required Storage Capacity (GWh) |
---|---|---|
2025 | 320 | 45,000 |
2030 | 890 | 210,000 |
The Storage Bottleneck: Why Current Tech Isn't Cutting It
Here's the problem in a nutshell: AI workloads aren't consistent. Training GPT-7 might require 500MW for 72 hours straight – that's like suddenly adding Las Vegas' power needs to the grid. Lithium-ion batteries? They're sort of the "plastic straws" of energy storage here – good for sipping, terrible for firehose demands.
- 60% efficiency loss in 4-hour discharge cycles
- 8-hour minimum recharge time for grid-scale systems
- $200/kWh storage cost (needs to hit $50 to be viable)
Three Breakthroughs Making Photovoltaic-Storage Systems AI-Ready
Wait, no – it's not all doom and gloom. 2024's Inflation Reduction Act extensions created a 30% tax credit for AI-aligned solar+storage projects. Combine that with these innovations:
1. Phase-Change Thermal Batteries (The "Molten Salt Miracle")
California's SolBank recently deployed thermal storage that holds 1.8GWh in molten silicon – enough to power 60,000 homes for a day. The kicker? It charges using excess solar from midday peaks.
"Our AI-driven predictive grid reduced storage waste by 40% last quarter." – SolarEdge CTO, April 2025
2. Self-Healing Perovskite Solar Cells
MIT's latest PV panels achieve 33% efficiency through machine learning-optimized material structures. Even better, they regenerate surface defects overnight. Kind of like Wolverine for solar tech.
3. Hydrogen Hybrid Systems
Germany's NEW 4.0 project combines:
- 500MW solar farm
- 100MW electrolyzers
- Underground salt cavern storage
Case Study: How Texas Avoided an AI-Induced Blackout
When Meta's new AI cluster in Austin spiked demand by 1.2GW last summer, ERCOT didn't fire up gas plants. Instead, they deployed:
- Distributed solar from 200,000 smart meters
- Vehicle-to-grid batteries from 18,000 Teslas
- Dynamic pricing algorithms adjusting every 90 seconds
The outcome? A 12% cost reduction compared to traditional peaker plants – and zero service interruptions.
The Roadmap: Where Do We Go From Here?
To hit 2030 targets, we need:
- AI-optimized microgrids (like Google's Project Sunroof 2.0)
- Standardized storage-as-a-service models
- Dynamic policy frameworks updated quarterly