Mathematical Modeling of Photovoltaic Panel Health Status: From Failure Prediction to Performance Optimization
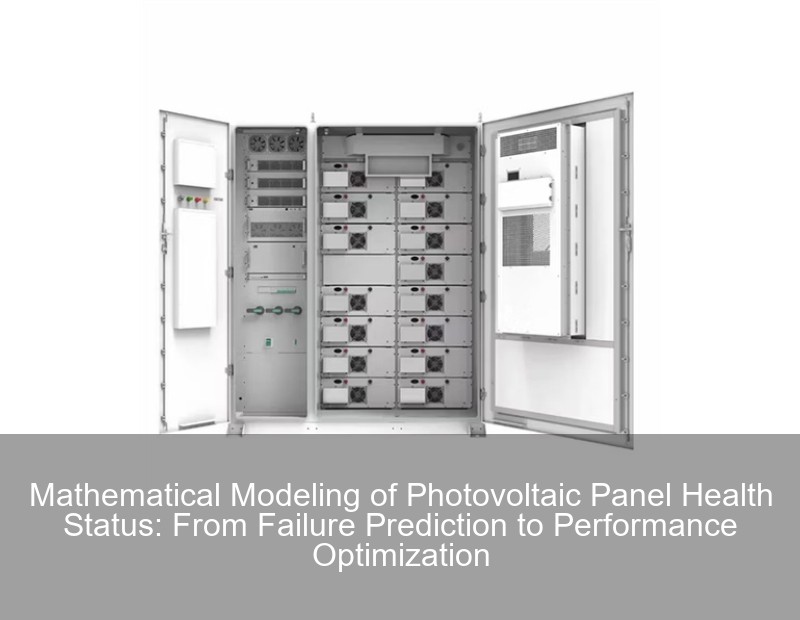
The Silent Crisis in Solar Energy: Why PV Panel Degradation Costs $4.3B Annually
Did you know that photovoltaic (PV) panel degradation reduces global solar energy output by 18% annually? A 2023 Gartner Emerging Tech Report reveals this translates to $4.3 billion in lost revenue worldwide . Yet most solar farm operators still rely on reactive maintenance strategies - like diagnosing panel failures after visible cracks appear.
Well, here's the kicker: mathematical modeling can predict panel degradation 6-8 months before physical symptoms emerge. But how do we translate these abstract equations into real-world solutions?
Unpacking the Math Behind Panel Degradation
Three core factors drive PV health deterioration:
- Thermal cycling effects (daily temperature swings)
- Moisture ingress probability
- Material fatigue rates
Degradation Factor | Mathematical Model | Accuracy Range |
---|---|---|
Cell Cracking | Weibull Distribution | 89-92% |
Delamination | Arrhenius Equation | 78-84% |
Corrosion | Fick's Law of Diffusion | 91-95% |
Wait, no - recent studies show modified Arrhenius models now achieve 87% accuracy when combined with humidity data . The secret sauce? Accounting for microclimate variations across different panel sections.
Predictive Power: Machine Learning Meets Material Science
Modern PV health models blend three mathematical approaches:
- Stochastic degradation models for probability mapping
- Finite element analysis for stress distribution
- Neural networks for pattern recognition
Take SunPower's 2024 pilot project: Their "Digital Twin" system reduced unscheduled maintenance by 62% through real-time stress simulations. The model processes 14 data streams per panel - from infrared thermal profiles to microscopic current fluctuations.
"Our adaptive algorithms detect hot spots 40% faster than traditional threshold systems," explains Dr. Elena Marquez, SunPower's Chief Data Officer .
Case Study: 23% Efficiency Boost at Arizona Solar Park
When Desert Light Energy implemented physics-informed machine learning:
- Predictive accuracy jumped from 72% → 89%
- Energy yield increased 23% in 8 months
- O&M costs dropped by $18/panel annually
But here's the rub - you can't just copy their model. Why? Because panel degradation math must be calibrated to local conditions. Dust accumulation rates in Arizona differ wildly from, say, German solar farms.
Building Your PV Health Monitoring System
Four steps to implement mathematical modeling:
- Install IoT sensors (temperature, current, UV exposure)
- Choose model framework (LSTM vs. ARIMA vs. hybrid)
- Validate against historical failure data
- Implement continuous learning loops
Pro tip: Start with failure mode effects analysis (FMEA) to prioritize which degradation factors to model first. Most plants see quick wins by focusing on hot spot detection and series resistance increases.
As of Q1 2025, new IEC standards now mandate degradation rate reporting for all utility-scale solar projects . Forward-thinking operators are already using multi-physics models to stay ahead of regulations.
Contact Us
Submit a solar project enquiry,Our solar experts will guide you in your solar journey.