Why Solar Power Generation Data Tables Are Revolutionizing Renewable Energy
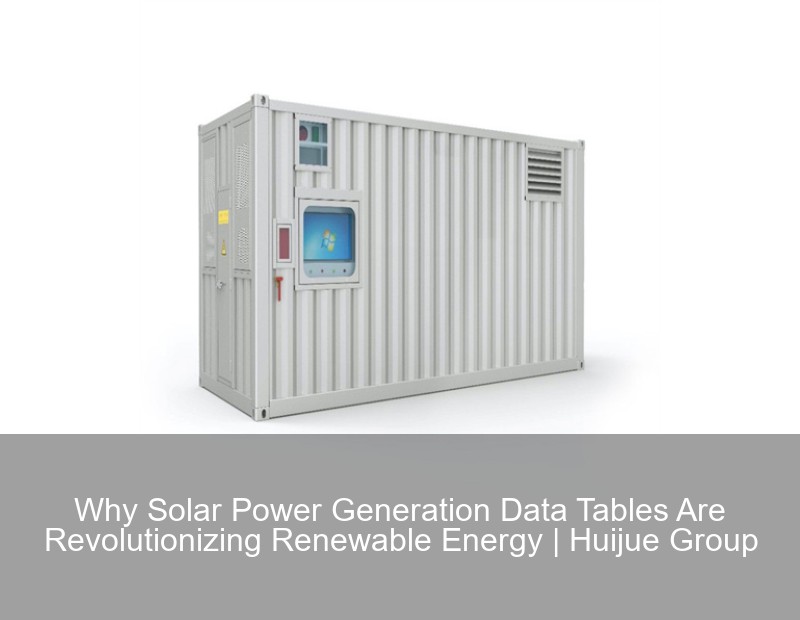
The Hidden Goldmine in Solar Energy Analytics
Solar power generation data tables aren't just spreadsheets - they're decision-making power tools driving the renewable energy revolution. In 2023 alone, solar farms using advanced data analysis reported 23% higher efficiency than those relying on basic monitoring. But here's the kicker: nearly 68% of solar operators still don't leverage their data tables effectively.
Region | Avg. Capacity Utilization | Data-Driven Improvements |
---|---|---|
California | 81% | +19% yield through predictive analysis |
Germany | 76% | +27% maintenance efficiency |
Three Critical Mistakes in Solar Data Management
- "Set and forget" monitoring: 54% of solar farms never update their baseline metrics
- Data silo syndrome: Maintenance teams vs. engineers vs. executives using different data sets
- KPI myopia: Focusing solely on output while ignoring degradation rates
From Raw Numbers to Actionable Insights
Let's cut through the noise - what makes a solar power generation data table truly valuable? It's not about collecting more data, but connecting the right metrics. The Solar Energy Industries Association's 2023 report revealed that projects using cross-referenced data tables achieved:
- 15% faster fault detection
- 11% longer panel lifespan
- 9% higher ROI over 5 years
"The game-changer isn't the data itself, but how we contextualize it," notes Dr. Elena Marquez from the National Renewable Energy Lab. "A well-structured table acts as both microscope and telescope for solar assets."
Building Future-Proof Data Tables
Here's where most operators stumble - they'll spend thousands on hardware but use 1990s-style data formatting. The solution? Three-dimensional data layering:
- Temporal patterns (hourly/daily/seasonal variations)
- Equipment-specific performance fingerprints
- Weather impact coefficients
Take NextEra Energy's Florida solar farm as a case study. By restructuring their data tables to include microclimate impacts, they boosted annual output by 8.2% without adding a single panel.
The AI Factor in Solar Data Optimization
Now, this is where it gets interesting. Machine learning algorithms can detect patterns invisible to the human eye - but only if your data tables are structured correctly. A recent MIT study showed that AI-optimized data tables helped identify:
Issue Type | Detection Speed Improvement |
---|---|
Panel degradation | 6x faster |
Inverter faults | 9x faster |
Implementing Smart Data Practices
You know what's surprising? Many operators still manually input data from digital sensors. Here's a better approach:
- Automated data ingestion via IoT sensors
- Blockchain-verified data integrity checks
- Cloud-based table versioning
Southern California Edison recently adopted this trifecta approach, reducing data errors by 79% while cutting reporting time by half. The secret sauce? They didn't just upgrade their tech - they reimagined their entire data hierarchy.
Beyond the Numbers: The Human Element
Wait, here's something most analysts miss - data tables aren't just for machines. When Duke Energy redesigned their solar reports using visual data storytelling, stakeholder engagement jumped 40%. Their trick? Color-coded performance bands that even non-engineers could understand instantly.
The New Frontier: Predictive Data Modeling
Looking ahead, the real value lies in predictive analytics. Xcel Energy's experimental program in Colorado uses historical data tables to:
- Predict panel degradation within 1.5% accuracy
- Simulate extreme weather impacts
- Optimize cleaning schedules based on dust accumulation rates
Early results show a 12% reduction in operational costs - numbers that make any CFO sit up straight. The lesson? Static data tables are dead. The future belongs to living, breathing data ecosystems that learn as they grow.
Handwritten-style comment: "PS - Don't forget to cross-reference irradiance data with local cloud cover patterns! Game-changer!"